Identification approaches for steel strip surface defects in hot rolling Process
Keywords:
Computer vision, Detection & Classification, Rolling process, Quality & Surface defectsAbstract
In steel manufacturing process, flat products are greatly concerned with the surface quality and the possibilities of its on-line
inspection. The visual control is obviously unable to continuously check the surface of the moving product, and the control at the ending stage remains not suitable although, it may provide information about process trends and parameters history. So, strip surface defects that are not detected yield to product downgrading or to costly rework operations for producer and/or end users. With such needed quality level, steel surface inspection systems are more and more implemented for detecting defects and allowing correction at appropriate time. Based on Computer vision, these applications make a use of detection and classification algorithms to identify these arising defects. The present work is related to a Project of a scientific and economic impact: The Development of an on-line inspection system for strip surface defects identification during the thermo-mechanical treatment in hot rolling process. We asses, in this work, some approaches in labeling each of the defects belonging to a database created for this aim. This Dataset is compound of five, among the most frequent, surface defect types and with 108 variants of each one. Obtained results shown the importance of the choice of a relevant image features extractor.
References
Neogi N., Mohanta D. K. and Dutta P. K., “Review of vision-based steel surface inspection systems”, EURASIP Journal on Image and Video Processing (2014), http://jivp.eurasipjournals.com/content /2014/1/50
Xie X., “A Review of Recent Advances in Surface Defect Detection using Texture analysis Techniques”, Electronic Letters on Computer Vision and Image Analysis 7 (3) (2008) 1-22, Published by Computer Vision Center / Universitat Autonoma de Barcelona, Barcelona, Spain
Wu G., Kwak H., Jang S., Xu K., Xu J., Design of Online Surface Inspection System of Hot Rolled strips (Proceedings of the IEEE International Conference on Automation and Logistics Qingdao, China, 2008), pp. 2291–2295
Caleb P., Steuer M., Classification of surface defects on hot rolled steel using adaptive learning methods, KES’2000, Fourth international conference on knowledge-based intelligent engineering systems and allied technologies. Proc. 1 (2000) 103–108
Shiyang Zhou, Youping Chen, Dailin Zhang, Jingming Xie, Yunfei Zhou, “ Classification of Surface Defects on Steel Sheet Using Convolutional Neural Networks”, MATERIALS AND TECHNOLOGY, doi:10.17222/mit.2015.335, (2016); at: https://www.researchgate.net/publication/ 313896846
Maoxiang CHU, Rongfen GONG and Anna WANG. “Strip Steel Surface Defect Classification Method Based on Enhanced Twin Support Vector Machine”, ISIJ International, 54 (1) (2014)119–124
Bhandari S. H., Deshpande S. M., Deshpande S. M., “A Simple Approach to Surface Defect Detection”, 2008 IEEE Region 10 , Colloquium and the Third International Conference on Industrial and Information Systems, Kharagpur, December 8-10, (2008), INDIA.
Doo-chul Choi, Yong-Ju Jeon, Jong Pil Yun, Sung Wook Yun, and Sang Woo Kim , An Algorithm for Detecting Seam Cracks in Steel Plates World Academy of Science, Engineering and Technology, International Journal of Mechanical, Aerospace, Industrial, Mechatronic and Manufacturing Engineering, 6 (12) (2012) 2835-2838
Yong-JuJeon, Doo-chul Choi, Jong Pil Yun, Changhyun Park, Homoon Bae, and Sang Woo Kim , Automated Inspection Algorithm for Thick Plate Using Dual Light Switching Lighting Method, International Journal of Electrical, Computer, Energetic, Electronic and Communication Engineering, 6 (12) (2012) 1475-1478
Chaitali Tikhe, Chitode J.S., Metal Surface Inspection for Defect Detection and Classification using Gabor Filter, International Journal of Innovative Research in Science, Engineering and Technology (An ISO 3297: 2007 Certified Organization), 3 (6) June 2014
Golub G.H., “The Generalized Eigenvalue Problem”, Lectures on Matrix Computation,Ph.D program of the Dipartimento di Matematica “Istituto Guido Castelnuovo”, Lecture No. 11, Roma, 2004.
Daniel L. Swets, John (Juyang) Weng, “Using Discriminant Eigenfeatures for Image Reteival”, IEEE Transactions on Pattern Analysis and Machine Intelligence, 18 (8) August 1996.
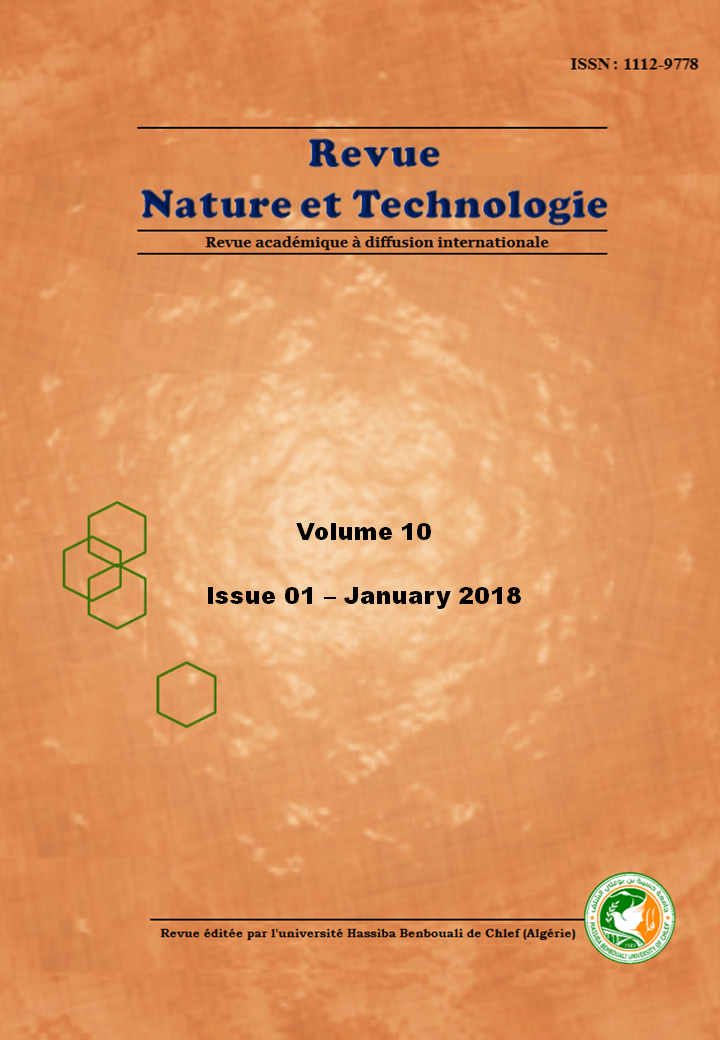
Downloads
Published
How to Cite
Issue
Section
License
Copyright (c) 2018 Nature & Technology Journal

This work is licensed under a Creative Commons Attribution 4.0 International License.
- All publications of "Nature & Technology Journal" are available under CC-BY Creative Commons Attribution 4.0 International which allows sharing, copying, reproduction, distribution, communication, reuse, adaptation by all means, in all formats and under all licenses.
- Any exploitation of the work or derivative works, including for commercial purposes, is possible. The only obligation is to credit the creators of the authorship of the original works, to indicate the sources and to indicate if modifications were made to the works (obligation of attribution).
This License gives:
- Nature & Technology Journal the right to develop, promote, distribute and archive the article set cited above (including, without limitation, the right to publish the work in whole or in part in any form whatsoever) and ensure the widest dissemination.
- The author (s) reserves the right to use all or part of this article, including tables and figures of his own works, providing that the appropriate recognition is given to the publisher as the holder of the copyrights, and the right to make copies of this article for its own use, but not for sale.